The much-needed and accelerated transition to renewable energy sources brings various challenges with it. One of these challenges is integrating these “variable” energy sources into the electricity grid without causing an upset in the system. Simply put, the existence of imbalance costs provides an incentive to the electricity traders to provide the best estimate of production they can. But how can one accurately predict how much a wind, solar or hydro plant will generate? Fortunately, we have sophisticated tools, methods, and, last but not least, data.
Renewable power plants have non-continuous sources such as wind, solar radiation, precipitation. When forecasting these variables, the science of meteorology comes into play.
Until a few centuries ago, only atmospheric measurements came to mind when talking about meteorology. Even scientists thought that it was impossible to make meteorological forecasts due to the chaotic structure of the atmosphere. The meteorological forecasting idea was viewed as fortune-telling by some scientists. However, many people believed that somehow this chaotic structure could be predicted. The difficulties faced by sailors in the 19th century revealed the need for meteorological forecasts quite clearly. The development of the telegraph enabled the rapid transmission of instant meteorological situations and made it possible to transmit instant alerts. This progress facilitated the rapid transmission of meteorological warnings.
Numerical weather forecasting systems, firstly developed by Vilhelm Bjerknes at the beginning of the 20th century, have reached a very advanced point today with the evolution of computer technologies. Today, accurate meteorological forecasts can be made for any specific place on Earth for every hour in the week ahead with multi-core supercomputers. These forecasts directly or indirectly touch the lives of the entire world population.
Forecasting the hourly wind speed, temperature, and wind direction are essential in the wind energy industry. Using these meteorological forecasts and combining them with the performance of the assets (wind turbines, solar panels, etc), one can predict how much power the power plants will produce each hour. Power generation companies have to make these estimates and inform the system operator so that the production and consumption across the network stay in balance, avoiding power failures and possible wide black-outs. In cases where renewable energy sources do not meet the consumption, the gap is filled with more continuous energy sources (“baseload” plants) such as natural gas and coal power plants (“baseload” plants). Conversely, when renewable energy sources produce excess power, the baseload plants are requested to shed load to keep the system in equilibrium. All these “corrections” the system operator makes lead to costs. Therefore, the system operator imposes an “imbalance cost” on the responsible party if the actual production of a plant differs from the estimated production, and the direction of the difference further exacerbates the imbalance already existing in the network. While the general concept and logic behind imbalance costs are the same, the actual calculation and implementation of the mechanism differ from market to market.
Let’s take a look at the country-specific imbalance costs of the UK in recent years. The balancing costs are on the rise in proportion to the power price volatility, with monthly balancing costs above £300 million for October ’21, which is more than double what it was the year before. The 2021- 22 costs are seen close to £3 billion. System imbalance prices have reached intra-day highs of £4,000/MWh during recent periods, mainly due to the cost of calling coal and gas-fired power generation during lulls in wind output.¹
In a different type of analysis, 25 randomly selected wind farms in Turkey were analyzed for 2021. The results are summarized in the chart below.
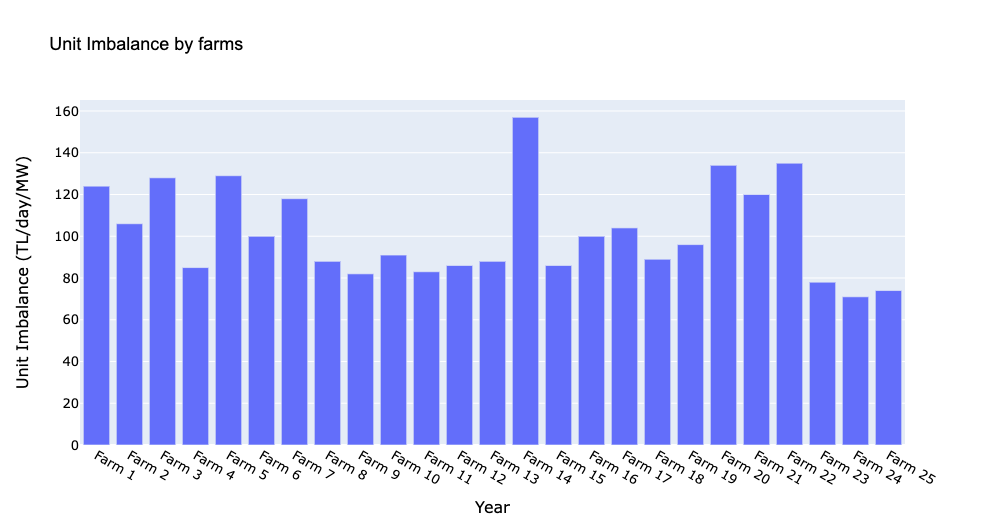
In 2021, the average PTF was around ₺500, and the average hourly unit imbalance cost (per MW) in the areas taken into calculation was approximately ₺5, with about 1-1.5% of potential gains lost as imbalance costs. Keep in mind that, with the increasing electricity prices, the monetary equivalent of these error rates increases and becomes more valuable. As explained above, the way to minimize the imbalance costs is to increase the production forecasts' accuracy, which can be quite tricky.
Kavaken offers the ultimate solution to increased imbalance costs. The Forecast+ module’s advanced analytics, which enables you to better forecast short-term production, helps reduce the imbalance costs to the lowest possible levels. Its unique approach benefits from combining the advantages of its machine learning model with additional input from commercially available forecast models. Kavaken uses the data from other forecast models and their accuracy levels in the past to ensemble them in a smart way to come up with the highest possible production forecast accuracy.
In addition to enabling more accurate forecasting with Forecast+, Kavaken’s predictive maintenance capabilities reduce unplanned shutdown and maintenance, which again has a positive impact on managing imbalance costs.
In summary,
- Imbalance costs are getting more attention with the increasing price of electricity
- There is available data that can be leveraged for managing imbalance costs
- Kavaken makes it easy to reduce imbalance costs
The methodology used for calculating the imbalance costs of the 25 farms in Turkey
The electricity price, forecast, and production data required for the analysis were obtained from the EXIST Transparency Platform.
Positive Imbalance Price (TL/MWH) = 0.97 * min(PTF, SMF)
Negative Imbalance Price (TL/MWH) = 1.03 * max(PTF, SMF)
Amount of Imbalance = Forecast - Production
(Production > Forecast):
Imbalance Income = Amount of Positive Imbalance * Positive Imbalance Price
(Production < Forecast):
Imbalance Loss = Amount of Negative Imbalance * Negative Imbalance Price
Day Ahead Income = PTF * Forecast
Total Income = Day Ahead Income + Imbalance Income - Imbalance Loss
Perfect Forecast Scenario = PTF * Production
Imbalance Cost = Perfect Forecast Scenario - Total Income
Unit Imbalance Cost = Imbalance Cost / Number of Days / Farm Production Capacity